The most advanced AI models possess hidden capabilities that extend far beyond what is immediately visible. In this article, we take a closer look at how you can uncover and leverage these inner functions to solve complex tasks that previously seemed impossible.
Generative AI models – and especially the large language models ChatGPT, Gemini and Claude – have for a few years now impressed people with their ability to cross established domains and create something new. It feels like true, creative intelligence when we get complex accounting concepts described in a Duckburg language, or when image generation models create new furniture inspired by large, soft cartoon teddy-bears.
This lack of limitation when they approach the edge of one domain, and the models instead infer across to other domains, is understandably incredibly fascinating. It is what allows the models to always respond, even when they are actually at the edge of their knowledge. They can guess the unsaid, jump to other areas of knowledge and create inference and purely language-statistical logical connections.
That is just not what creates the truly significant productive impact when the technology is put to work for us.
Breadth and depth
Fundamentally, you can view the (very) large models based on two parameters: breadth and depth. While the breadth of the models' training data and training has been the decisive factor in most initial attempts and implementations, and also what has created the aforementioned fascination, it has been quite overlooked ‒ or considered trivial ‒ how deeply the models are also trained within specific areas.
The challenge here has been that we have had to consider the depth as trivial because we have not been able to verify it ‒ we do not know the training data, so we do not know what and how much of this or that the models are trained on. In this way, our approach to them has been more like asking an enigma, where the breadth and the quirky connections became intriguing.
Nevertheless, the models have first achieved enormous significance partly due to their depth training: coding. Developers have embraced the large language models to an extent that we cannot imagine going back. Because, the models are trained on enormous amounts of code data, so they have an incredibly deep understanding of code.
What does a language model understand?
The question is therefore: ”What else do the models understand?
The tech company Anthropic, which is backed by Amazon and develops the language model Claude, which several people currently consider to be perhaps even better than ChatGPT, released a major study in early summer, where they had investigated which functions (features, groupings, connections) are found in a language model's brain.
By running the model in a particular way over millions of times, they were able to identify functions that, for example, pertained to the Golden Gate Bridge. There was a lot of knowledge associated with the bridge ‒ red, San Francisco, concrete etc. ‒ and this meant that the model had a linguistic concept of what the Golden Gate Bridge was. A feature, as they call it.
By intensifying this feature ‒ i.e. using code to increase the likelihood that the model would use Golden Gate Bridge in its responses ‒ they also experienced that the model began to behave differently than before. For instance, it started to believe that it was a large red bridge and not a language model. And there were countless of these functions hidden in the model. The existence of these functions means that there is hidden expertise within the models. And it is this expertise we need to uncover and build with.
For example, the models also have a function that pertains to invoices. They have seen so many different invoices that they know what they look like and also how an outlier invoice can be interpreted as an ordinary one ‒ there are logics within the models that can rationalize in a particular way that this is a customer number, this is a contact person etc. Similarly, they can also see what is an auto-signature in an email about, for example, an address change, so that two addresses in the same email are not confused. Because they also have a function that pertains to email structures and formats.
By uncovering these functions, predictability is also achieved. For example, try asking ChatGPT to complete this sentence: 'To be or not to be; that is ...', and you will always get 'the question'. But if you instead ask it to complete this sentence, you will get chaotic responses every time: 'The CFO went to Mars because ...'. Simply because there it uses its breadth to guess at something it does not really have the depth for. There is a Hamlet function hidden in a language model, but not an astronaut-CFO.
Identifying functions is a game changer
When we prompt engineer – that is design questions and tasks for an AI model – we always strive to frame the task and context. That is the crux of it. And we do this because we do not want it to respond broadly, but rather delve into a deeper context. Developers who use the models understand this intuitively, but it also needs to be understood on an analytical idea level.
The tasks we assign to a language model must therefore be both definable, so that a function/feature can be identified or dismissed, and fundamentally well-known, so we can expect that the models may have a function/feature that will serve our purpose.
This also means that we need to briefly set aside the chatbot that can answer everything and instead seek to uncover precise functions that can solve known problems. Instead of AI agents, which are currently a hot topic in the AI market, the real benefit for most companies lies in defining and developing AI roles. The difference is simply that an agent is expected to do everything you ask and be autonomous, whereas a role only takes the stage at the right time and with precise lines.
By defining the role, we regain predictability and measurable task solutions. We also gain the ability to use the models for what we have never been able to solve so easily with technology before: structuring the unstructured. Through roles built on identified functions, which can contextualize and logically interpret various inputs, a language model can bring order to otherwise arbitrary data. And that is the real game changer when it comes to the new wave of AI, as it expands the entire digital playing field.
The short message: By focusing the large AI models, you gain concrete benefits
After a few years of testing and spreading the message about AI within organisations, companies now face the challenge of creating real value with generative AI. The truth is that while the models initially impressed by resembling a C-3PO that could answer everything, the real benefit lies in identifying the models' inner functions and constraining them around these functions. The models are extremely knowledgeable, but the interesting part is discovering how much they know about one subject and one task, rather than how broadly they can spread over irrelevant areas.
Treat them like an employee to be hired: The person's knowledge of specific tasks is always more important than knowledge outside of them. Identifying precise (often unstructured) tasks and testing the models' accuracy on these is therefore crucial for deriving value from the large language models.
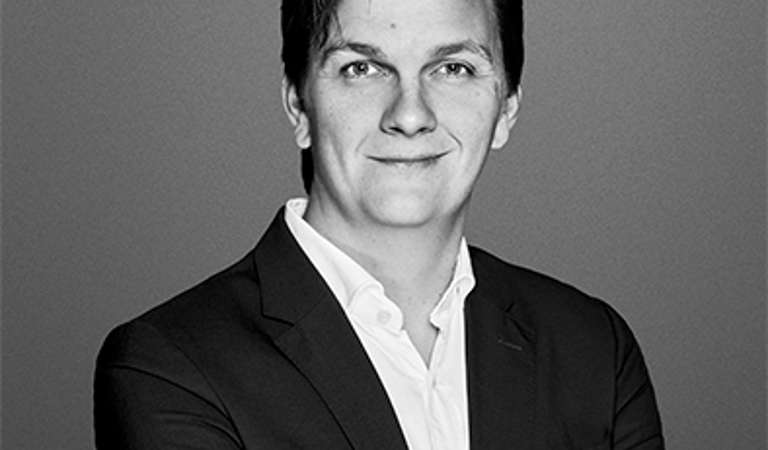
Do you want to understand more about both the depth and breadth of generative AI?
Then give our AI Lead, Lasse Rindom, a call. His engaging talks are sure to leave you with plenty of AI food for thought. And if you are looking for inspiration on how to use and implement generative AI to fit your company's purpose, vision and strategy, he is the person you are looking for.